The Ultimate Guide to AI Governance Standards
What Businesses Need to Know in 2025
04/12/2024
20 MIN READ
As AI transforms industries and reshapes business landscapes, organizations face a dual challenge: harnessing its immense potential while ensuring responsible and ethical deployment. At code4thought, we understand that achieving this balance requires not only adherence to global standards but also the practical tools to implement them effectively.
This consolidated guide to AI standards—spanning ISO 42001, ISO 25059, NIST AI RMF, ISO/IEC TR 29119-11, and OECD AI Principles—serves as your roadmap to navigating the complexities of AI governance. Each framework offers unique insights into critical areas such as risk management, fairness, explainability, security, and reliability. By comparing these standards and aligning them with the EU AI Act, we provide actionable guidance for organizations to make informed decisions tailored to their specific needs.
OECD AI Principles
Amidst the transformative wave of AI invoked in the digital ecosystem, the Organization for Economic Cooperation and Development (OECD) has introduced a comprehensive set of AI principles, a framework aimed at guiding responsible and effective AI adoption.
The OECD AI Principles are structured around five core principles and five recommendations for national policies and international cooperation.
Inclusive Growth, Sustainable Development, and Well-being
AI should contribute to broad societal benefits, fostering inclusive growth and sustainable development. This principle emphasizes that AI technologies should benefit everyone and advance civilization. It emphasizes the need to use AI to decrease disparities and promote sustainability, ensuring that all demographics and regions benefit from AI.
The principle involves creating AI-driven solutions that address global challenges such as climate change, healthcare, and education. By concentrating on these areas, companies can guarantee that their AI initiatives benefit all stakeholders. This can include developing AI apps that promote sustainability, improve healthcare, and educate marginalized areas.
Human-Centered Values and Fairness
To ensure fairness and equity, AI systems must respect human rights, dignity, and democracy. This principle emphasizes the relevance of human rights in AI technology development. AI systems should protect rights, avoid discrimination, and promote equality.
Adhering to this principle requires AI algorithm bias prevention policies and processes. AI applications must not reinforce prejudices or create new forms of discrimination. This requires diverse training data to appropriately reflect demographic groups, bias-free algorithms, and regular audits to assure fairness.
By doing so, businesses can develop AI solutions that are not only effective but also equitable, fostering trust and acceptance among users and stakeholders.
Transparency and Explainability
AI operations should be clear and explainable to people. AI processes should be transparent and visible to users and stakeholders so they can see how an AI system functions and makes decisions. Explainability includes clarifying the reasoning and logic behind AI decisions, which is crucial to establishing trust and validating AI outcomes.
Use this approach by thoroughly documenting AI decision-making processes and explaining them to users. AI implementations must be transparent and user-friendly to assist stakeholders in comprehending AI decisions.
Companies should build methods for resolving AI decision questions and concerns to promote trust in AI systems. Finally, businesses may boost user acceptance and happiness by stressing openness and explainability in their AI apps.
Robustness, Security, and Safety
AI systems should be secure and resilient throughout their lifespan. AI systems must be designed and operated with robustness and safety in mind. This includes protecting AI systems from cyberattacks, ensuring they work reliably, and adding fail-safes to reduce risks and prevent malfunctions.
Implementing this principle requires AI-specific cybersecurity best practices. This involves regular stress tests to determine the system’s ability to tolerate pressures and unforeseen events without affecting safety or performance. Such stress tests should include performance and trustworthiness (e.g. bias, explainability analysis) testing as well as evaluation against integrity violation and evasion attacks in accordance with ISO 29119-11.
Constant monitoring and regular updates maintain AI applications’ robustness and ensure that the developed AI systems will be secure, durable, and able to execute consistently and reliably, even when unexpected issues arise.
Accountability
Οrganizations and individuals responsible for AI systems should be held legally, ethically, and operationally accountable for their proper functioning and adherence to established principles. Accountability ensures that developers and managers of AI systems are responsible for their impact and that all actions and outcomes related to AI are properly managed and scrutinized.
Applying this principle requires clear AI governance structures with roles and duties. This can include committees dedicated to monitoring AI operations, implementing audit trails to oversee decision-making, and ensuring compliance with laws and regulations.
Additionally, businesses must develop mechanisms for addressing grievances and correcting errors, ensuring that issues related to AI systems are promptly addressed and effectively resolved.
OECD Recommendations for Trustworthy AI Adoption
The OECD paper also provides the following list of actionable recommendations:
- Invest in AI Research and Development: Encourage innovation while addressing ethical and technical challenges.
- Foster a Digital Ecosystem for AI: Promote policies that support data access, infrastructure, and skills development.
- Shape an Enabling Policy Environment for AI: Develop legal and regulatory frameworks that facilitate AI adoption while protecting public interests.
- Build Human Capacity and Prepare for Labor Market Transformation: Equip the workforce with the necessary skills to thrive in an AI-driven economy.
- International Cooperation for Trustworthy AI: Collaborate globally to address cross-border AI issues and establish common standards.
ISO/IEC 42001
ISO/IEC 42001 (ISO 42001) is the first AI Management System Standard devised by the International Organization for Standardization (ISO) and the International Electrotechnical Commission (IEC). The standard is designed to provide a comprehensive structure for organizations to manage AI systems responsibly.
Overview and Objectives
Published on December 18, 2023, ISO 42001 marks a pivotal step in standardizing AI management. As AI technologies become integral to business strategies, a consistent and transparent approach to managing these systems is critical. ISO 42001 addresses this need by establishing guidelines that help organizations design, develop, implement, deploy, and maintain AI systems that are responsible, reliable, and aligned with business objectives.
While powerful, AI systems have inherent risks such as bias, lack of accountability, and potential misuse. The voluntary ISO 42001 provides a framework for identifying, assessing, and mitigating these risks based on transparency, accountability, bias identification and mitigation, safety, and privacy. This proactive approach protects the organization and safeguards users and society from unintended consequences of AI deployment.
One of ISO 42001’s primary objectives is to build trust in AI systems. With the EU AI Act being enforced since 1 August 2024, organizations can demonstrate their commitment to responsible AI practices by adhering to ISO 42001, thereby enhancing stakeholder confidence and fostering a culture of transparency. Moving beyond compliance, ISO 42001 can enable businesses to experience the increased business value of trustworthy AI.
Adopting a standardized approach to managing AI systems is strongly recommended over implementing a custom or do-it-yourself approach. Following established standards and best practices can help ensure consistency, reliability, and compatibility across various AI systems and tools in an organization’s ecosystem. The Standard is structured around the “Plan-Do-Check-Act” process for establishing, implementing, maintaining, and continually improving artificial intelligence. This approach is crucial as it ensures that the value of AI for growth is acknowledged and the appropriate level of oversight is in place.
The Standard applies to any organization, based on the official faqs: “Organizations of any size involved in developing, providing, or using AI-based products or services. It is applicable across all industries and relevant for public sector agencies as well as companies or non-profits.”
Structure and Key Components of ISO 42001
Like other Standards, ISO 42001 includes a number of annexes that offer businesses comprehensive advice and support, such as AI system development management guidance, a list of AI controls and how to implement them, organizational goals and sources of risk related to AI, and sector-specific guidelines.
ISO 42001 addresses various aspects of the AI system lifecycle, starting from the early concept phase and extending to the system’s ultimate deployment and operation. One of the standard’s essential requirements is leadership. Top management must exhibit strong leadership and unwavering dedication to the AI management system (AIMS). They should set forth policies and objectives that align with the organization’s strategic vision.
Strategic planning is another essential element. Organizations must recognize and evaluate potential risks and opportunities linked to AI and create a comprehensive strategy to tackle them. In terms of support, organizations must offer resources and assistance for the AIMS, including training, awareness, and communication initiatives.
Efficient and effective processes and procedures must be established to develop, deploy, and maintain AI systems. Performance evaluation requires continuous monitoring, measurement, analysis, and assessment of AI systems to make necessary adjustments.
Finally, ISO 42001 emphasizes the importance of continuous improvement, consistently enhancing the AIMS to ensure its ongoing relevance and effectiveness.
Implementing the Standard
For business leaders, the implementation of ISO 42001 can seem challenging. However, a structured approach can simplify the process and ensure successful adoption. Here is a step-by-step guide to implementing ISO 42001 in your organization:
- Conduct a Readiness Assessment: Evaluate your organization’s current AI capabilities and identify gaps that must be addressed. Review existing policies, processes, and resources related to AI management.
- Establish Governance Structures: Create governance structures to oversee AI initiatives. Form an AI governance board or committee, assign roles and responsibilities, and define decision-making processes. Ensure that senior leadership is involved to drive commitment and accountability.
- Develop an AIMS: Integrate AIMS with existing organizational processes, ensuring continuous improvement and alignment with ISO standards.
- Develop a Risk Management Framework: Develop a comprehensive framework tailored to your organization’s AI applications. Identify potential risks, assess their impact, and establish mitigation strategies. Regularly review and update the framework to address new risks as they emerge.
- Implement Data Management Practices: Ensure robust data management practices; establish data quality, integrity, and privacy protocols. Implement measures to comply with data protection regulations and regularly audit data processes to maintain compliance.
- Enhance Algorithmic Transparency: Focus on enhancing the transparency and explainability of your AI models. Provide clear, understandable explanations of how outcomes are generated. Engage with stakeholders to ensure that they comprehend and trust the AI systems.
- Embed Responsible Principles: Incorporate trustworthy and responsible principles into every AI development and deployment phase. Conduct impact assessments to evaluate your AI systems’ societal and human rights implications. Establish mechanisms to ensure ongoing compliance.
- Educate your Personnel: Invest in training programs, like AI ethics, risk management, data management, and algorithmic transparency, to equip your staff with the knowledge and skills needed to implement and maintain ISO 42001.
Finally, all processes must be documented, and the external audit conducted by an accredited body must be successfully passed to get certified for three years with annual surveillance audits.
ISO/IEC 25059
The rapid adoption of AI across industries demands not only innovative solutions but also rigorous governance to ensure systems operate reliably, fairly, and transparently. ISO 25059: Quality Requirements and Evaluation (QRE) for AI Systems was developed to address these critical needs, providing a structured framework for assessing and enhancing the quality of AI systems throughout their lifecycle.
Key Objectives of ISO 25059
ISO 25059 establishes standardized quality attributes tailored specifically for AI applications. The standard ensures that AI systems meet technical, operational, and ethical benchmarks, making them fit for purpose in high-stakes or consumer-facing environments. The overarching goal is to align AI development and deployment with organizational objectives, user needs, and societal expectations.
Core AI Quality Attributes
ISO 25059 provides a robust framework for evaluating the quality of AI systems through two complementary models: the Product Quality Model and the Quality in Use Model. Each model focuses on distinct but interconnected attributes that ensure AI systems meet technical, operational, and ethical standards across their lifecycle.
1. Product Quality Model
The Product Quality Model in ISO 25059 provides a comprehensive framework for evaluating the intrinsic quality attributes of AI systems. These attributes are categorized into eight key dimensions, ensuring that AI systems are designed to meet functional, operational, and security requirements while remaining adaptable to changing environments.
- Functional Suitability: This dimension ensures the AI system fulfills its intended purpose effectively, emphasizing on correctness and adaptability. Functional Correctness ensures the system produces outputs that are consistent with its defined objectives and requirements, minimizing errors, while Functional Adaptability is the AI system’s ability to adapt to different operational environments or scenarios without requiring significant reconfiguration.
- Performance Efficiency: Evaluates the system’s efficiency in utilizing resources while maintaining performance.
- Compatibility: Measures the AI system’s ability to coexist and interact with other systems.
- Usability: Focuses on the ease of use and user-centric design of the system. For AI systems, the two essential characteristics are Controllability and Transparency. Controllability is the extent to which the system allows users to control and intervene in its operations when necessary, ensuring safety and accountability, while Transparency focuses on providing clear, interpretable, and accessible explanations of the AI system’s decisions and processes.
- Reliability: Ensures consistent operation under various conditions. For AI systems, emphasis is placed on Robustness. This attribute evaluates the system’s resilience to handle unexpected inputs, adversarial attacks, or system failures without compromising functionality.
- Security: Focuses on protecting the system and its data from threats. Besides typical attributes like confidentiality and integrity, Intervenability is crucial for AI systems. This is the ease with which human operators can modify or override the system’s behavior when needed to maintain control and prevent harm.
- Maintainability: Addresses the system’s ability to be updated and improved over time.
- Portability: Evaluates the ease of transferring the system across environments.
2. Quality in Use Model
The Quality in Use Model evaluates the system’s performance and impact in real-world contexts, focusing on the end-user experience and societal outcomes. It emphasizes:
- Freedom from Risk: Addresses the societal and ethical risks associated with deploying AI systems, ensuring that they do not cause harm to users, organizations, or society. For AI systems, the standard emphasizes the need for Societal and Ethical Risk Mitigation. This includes considerations such as accountability, fairness and non-discrimination, transparency, and privacy. By addressing themes like human-centered design, professional responsibility, and respect for international norms, this attribute ensures AI systems align with societal expectations and promote positive human values. Organizations must evaluate how their AI systems affect community involvement, labor practices, and adherence to the rule of law, fostering trust and sustainable adoption of AI technologies.
- Transparency in Use: Ensures that end-users understand how the system operates, enabling trust and informed decision-making.
These two models work in tandem to deliver a comprehensive approach to quality assurance. The Product Quality Model focuses on internal system attributes, while the Quality in Use Model ensures the AI system performs effectively, ethically, and safely in its deployment context.
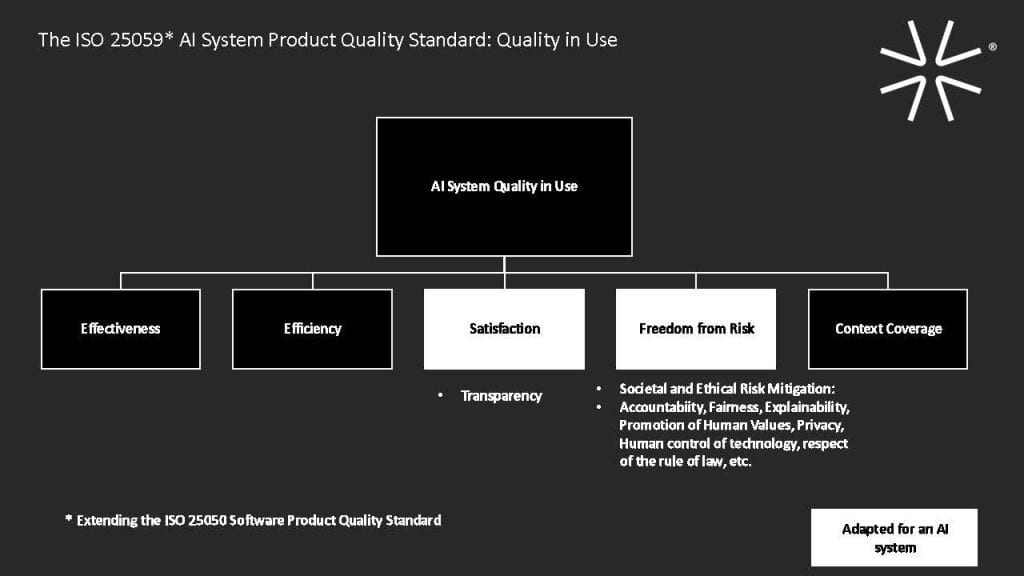
Applicability and Benefits
ISO 25059 is particularly relevant for industries where the quality of AI systems has direct implications for safety, trust, and performance, such as healthcare, finance, autonomous systems, and public services. By offering a comprehensive evaluation framework, it allows organizations to:
- Identify and address quality gaps early in the development cycle.
- Enhance trust among stakeholders, including users, regulators, and the public.
- Streamline compliance with regulatory standards, such as the EU AI Act or OECD AI Principles.
Complementary Role in AI Governance
ISO 25059 works effectively alongside other AI governance standards. For instance:
- It complements ISO 42001, which focuses on AI management systems, by providing measurable quality benchmarks to operationalize governance frameworks.
- It aligns with NIST AI RMF, emphasizing robustness and risk mitigation.
- It strengthens ISO/IEC TR 29119-11 by offering additional evaluation criteria for testing AI systems.
- It shares a commitment to fairness and transparency with the OECD AI Principles, translating these values into actionable metrics.
Implementation of ISO 25059: Practical Steps for Businesses
Adopting ISO 25059 is a pathway to building trustworthy, high-quality AI systems that deliver consistent results while aligning with ethical and societal expectations. Implementing this standard requires careful planning, cross-functional collaboration, and a commitment to continuous improvement. Below are the key considerations and steps businesses should take to implement ISO 25059 effectively:
1. Understand the Standard’s Requirements
Before implementation, businesses should thoroughly understand the quality attributes defined by ISO 25059. This requires reviewing the standard’s documentation and aligning its principles with organizational goals.
- Tip: Assign a dedicated team to interpret and map ISO 25059 requirements to your organization’s specific AI use cases.
2. Assess Current AI Systems
Conduct a comprehensive audit of existing AI systems to identify gaps in quality, performance, and governance. Use ISO 25059 as a benchmark to evaluate areas like robustness, bias mitigation, and explainability.
- Consideration: Leverage tools such as code4thought’s iQ4AI platform to automate and streamline this assessment, ensuring consistency and depth in your evaluations.
3. Establish Cross-Functional Teams
Implementing ISO 25059 requires collaboration across technical, operational, and ethical domains. Establish a cross-functional team comprising data scientists, developers, quality assurance professionals, legal advisors, and ethics officers.
- Tip:Appoint a dedicated AI governance lead to oversee the standard’s implementation and integration into broader business processes.
4. Define Quality Metrics and KPIs
Set clear, measurable quality metrics aligned with ISO 25059 attributes. For example:
- Functional Correctness: Accuracy of predictions within predefined error margins.
- Transparency: Percentage of explainable model outputs.
- Fairness: Equity metrics based on demographic outcomes.
- Consideration: Use tools and platforms that offer advanced analytics and visualization capabilities to track these KPIs over time.
5. Integrate Quality Assurance into the AI Lifecycle
Embed ISO 25059 requirements into each stage of the AI lifecycle, from design and development to deployment and monitoring. Key focus areas include:
- Bias mitigation during data preparation and model training.
- Reliability testing under diverse scenarios and stress conditions.
- Transparency reviews to ensure outputs are explainable and interpretable.
6. Develop Documentation and Reporting Practices
Document all processes, decisions, and evaluations related to AI quality. This not only ensures compliance with ISO 25059 but also supports transparency with regulators and stakeholders.
- Tip: Use reporting templates that align with other standards like the EU AI Act for seamless compliance across frameworks.
7. Foster a Culture of Continuous Improvement
AI systems evolve over time as new data, threats, and use cases emerge. Businesses must establish a process for regularly re-evaluating systems against ISO 25059’s benchmarks and updating them as needed.
- Consideration: Conduct periodic quality audits and user feedback sessions to identify areas for improvement.
8. Align with Complementary Standards
Leverage ISO 25059 in conjunction with related standards like ISO 42001 (AI management systems), ISO/IEC TR 29119-11 (AI system testing), or NIST AI RMF (risk management). ISO 29119-11’s emphasis on rigorous testing methodologies complements ISO 25059’s quality evaluation framework, ensuring that AI systems are not only built to meet performance and reliability requirements but are also thoroughly validated against diverse scenarios. This ensures a holistic approach to AI governance, balancing quality, risk, and ethics.
NIST AI Risk Management Framework (AI RMF)
To address the risks and challenges of AI adoption and use, the National Institute of Standards and Technology (NIST) has developed the AI Risk Management Framework (AI RMF), a voluntary framework designed to help organizations manage the risks associated with AI technologies.
Recognizing the need for a standardized approach to AI risk management, NIST initiated a collaborative effort involving industry, academia, government, and civil society stakeholders. This collaborative approach ensured the framework would be robust, comprehensive, and adaptable to various contexts and industries.
The NIST AI RMF aims to promote the development and use of AI in a way that is responsible, trustworthy, and aligned with societal values. It provides a structured approach around four core functions to identify, assess, and mitigate the potential risks AI systems pose.
The Framework’s Core Functions
Map
The Map function serves as the foundation for effective AI risk management. It involves a comprehensive analysis of the AI system’s ecosystem, including its purpose, design, and potential impacts. Organizations must clearly define the system’s objectives and intended use cases and identify all relevant stakeholders. This function also requires a thorough understanding of the AI system’s technical capabilities, limitations, and dependencies.
A crucial aspect of the Map function is the identification of potential risks across various dimensions, including technical, ethical, societal, and legal considerations. This involves anticipating how the AI system might fail, be misused, or produce unintended consequences. The Map function also emphasizes the importance of considering the broader context in which the AI system will operate, including cultural, regulatory, and industry-specific factors.
Measure
The Measure function quantifies and evaluates the AI system’s performance, reliability, and impact. This involves developing and implementing robust testing methodologies and metrics to assess the system’s behavior under various conditions. Key areas of measurement include:
- Data quality and representativeness.
- Model accuracy and fairness
- System robustness and security
- Explainability and interpretability of AI decisions
- Compliance with ethical guidelines and regulatory requirements
The Measure function also emphasizes the importance of continuous monitoring and evaluation throughout the AI system’s lifecycle. This includes tracking performance drift, identifying emerging risks, and assessing the system’s long-term impact on individuals and society.
Manage
The Manage function is centered on implementing effective strategies to address and mitigate the risks identified in the Map and Measure phases. This involves developing and deploying comprehensive controls, policies, and procedures tailored to the specific AI system and its associated risks. Key aspects of the Manage function include:
- Implementing technical safeguards and fail-safe mechanisms
- Developing clear operational guidelines and best practices
- Establishing incident response and error correction processes
- Ensuring proper data management and privacy protection
- Implementing version control and change management procedures
The Manage function emphasizes the importance of adaptability and continuous improvement. As new risks emerge or the AI system evolves, organizations must be prepared to adjust their management strategies accordingly.
Govern
The Govern function focuses on establishing and maintaining oversight structures to ensure responsible AI development and deployment. This function goes beyond technical considerations to address organizational, ethical, and societal aspects of AI risk management. Key components of the Govern function include:
- Developing a clear AI governance structure with defined roles and responsibilities
- Establishing ethical guidelines and decision-making frameworks
- Ensuring compliance with relevant laws, regulations, and industry standards
- Implementing transparency measures to build trust with stakeholders
- Fostering a culture of responsible innovation and ethical AI use
The Govern function also emphasizes the importance of stakeholder engagement, including mechanisms for feedback, dispute resolution, and accountability. It requires organizations to regularly review and update their governance practices to keep pace with evolving AI technologies and societal expectations.
ISO/IEC TR 29119-11
ISO/IEC TR 29119-11 is a standard dedicated to testing AI-based systems, offering numerous benefits for businesses via a structured and universally recognized approach to AI software testing.
Overview of ISO/IEC TR 29119-11
ISO/IEC TR 29119-11 is actually a technical report and part of the broader ISO/IEC 29119 series of standards, which provides guidelines and best practices for software testing across various phases of the software development lifecycle.
TR 29119-11 focuses on and addresses the unique challenges associated with AI-based systems testing. Unlike traditional software, AI systems learn and evolve continuously, making their behavior less predictable and more complex to test. ISO/IEC TR 29119-11 provides guidelines and best practices to ensure these systems perform as intended and mitigate all associated risks.
The inception of ISO/IEC TR 29119-11 was rooted in late 2020 in the growing need for standardized testing methodologies for AI systems. As AI technologies began to diffuse through various industries – from self-driving cars and smart vacuums to checkout-free grocery shopping and machine learning for healthcare – stakeholders recognized the absence of comprehensive testing standards.
This gap led to collaborative efforts among international experts in AI and software testing, culminating in the development of ISO/IEC TR 29119-11, which forms a consensus on the best practices required to ensure the quality and reliability of AI systems.
ISO/IEC TR 29119-11 is meticulously structured to cover all facets of AI system testing. Its key components include:
- Scope: Outlines its purpose and its applicability to different types of AI systems.
- Concepts: Defines key terms and concepts to ensure a common understanding among practitioners.
- Planning: Provides guidance on planning tests for AI systems, considering factors such as system complexity, learning mechanisms, and operational environment.
- Design: Discusses methodologies for designing effective tests, including the selection of appropriate test data and scenarios.
- Execution: Covers the execution of tests, including monitoring system behavior, logging results, and handling anomalies.
- Evaluation and Reporting: Offers guidelines for evaluating test results and reporting findings to stakeholders.
- Further Considerations: Addresses the ethical and legal implications of testing AI systems, emphasizing the need for transparency and accountability.
Implementation of the Standard
Implementing ISO/IEC TR 29119-11 requires a systematic approach. The process begins with a gap analysis, where current testing practices are assessed against the standard to identify gaps and areas for improvement. Following this, and to maximize the standard’s guidelines efficacy, educating the testing team and relevant stakeholders on the standard’s requirements and best practices is crucial to ensure everyone involved is aware of the necessary steps.
The next steps are developing comprehensive test plans and designing test cases that align with the standard’s regulations. Appropriate tools for test automation, data management, and result analysis are then identified and deployed to facilitate the testing process.
At this point we need to underline the fact that the standard merely provides guidance on what aspects to test and indicatively provides ways/approaches for doing those tests. It is up to the teams/organization to decide the “how”. For instance, in the case of Bias Testing, the standard suggests the use of expert reviews of datasets, which can be time-consuming and an error-prone process. In order to implement this test, our team at code4thought has automated the task by using industry-accepted metrics such as the Disparate Impact Ratio.
During the actual testing phase, tests are executed rigorously, AI system behavior is monitored, and outcomes are meticulously logged for further analysis. The final phase involves evaluating the test results, documenting findings, and communicating them to stakeholders to ensure transparency and informed decision-making.
Comparative Analysis of AI Standards
The rise of AI governance has led to a diverse ecosystem of standards, each with a unique focus, methodology, and regional context. While each standard provides a structured approach to responsible AI, understanding their specific emphases is crucial for organizations seeking to apply them effectively. This section compares the five major standards—ISO 42001, ISO 25059, NIST AI RMF, ISO/IEC TR 29119-11, and the OECD AI Principles—alongside the EU AI Act.
Core Focus Areas Across Standards
Each standard addresses key governance issues such as model performance, fairness, explainability, robustness, and reliability, but with varying depth and approaches:
ISO 42001 focuses on management systems for AI, offering organizations a structural framework to integrate AI governance into their broader operations. This standard prioritizes management oversight and compliance pathways that align with broader corporate governance.
ISO 25059 provides a structured approach to evaluating AI quality characteristics, including functionality, fairness, and explainability, ensuring systems meet user and societal expectations.
NIST AI RMF emphasizes risk assessment and mitigation specifically for AI-related threats. It takes a systematic approach to identifying, assessing, and managing AI risks, particularly targeting security and robustness, to address concerns about resilience against attacks and malfunctions.
ISO/IEC TR 29119-11 is dedicated to AI testing protocols, providing technical guidelines for assessing AI model quality and reliability. It covers testing methodologies to evaluate model accuracy, robustness, and performance under various conditions, which is critical for industries reliant on high-stakes AI decisions.
OECD AI Principles highlight ethical AI deployment with an emphasis on transparency, fairness, and accountability. They are less prescriptive but encourage a framework that ensures responsible adoption, advocating for human-centered AI that respects privacy and fairness.
The key differences between the five standards can be summarized as follows:
- Rigidity vs. Flexibility:
ISO 42001 and ISO/IEC TR 29119-11 are stringent in protocol and process adherence, making them suited for highly regulated industries. NIST AI RMF, OECD AI Principles, and ISO/IEC 25059 allow varying degrees of flexibility, providing frameworks adaptable across a range of risk profiles and industry needs.
- Quality Assessment:
ISO/IEC 25059 uniquely provides a standardized approach to quality assessment for AI systems, introducing AI-specific quality attributes like functional adaptability and robustness. It extends existing software quality standards to address AI systems’ unique characteristics.
- Ethics and Societal Impact:
The OECD AI Principles uniquely address ethical concerns and societal impacts, prioritizing values like fairness and transparency. While other standards focus primarily on operational and technical reliability, ISO/IEC 25059 incorporates some ethical considerations through characteristics like transparency and user controllability.
- Risk Management Approaches:
NIST AI RMF provides a granular, technical risk management framework. ISO/IEC 25059 complements this by offering a standardized quality model that can support risk assessment and mitigation strategies for AI systems.
- Implementation:
ISO 42001 and ISO/IEC 25059 offer potential for certification, while NIST AI RMF and OECD AI Principles are voluntary frameworks. ISO/IEC TR 29119-11 provides technical guidance without a certification component.
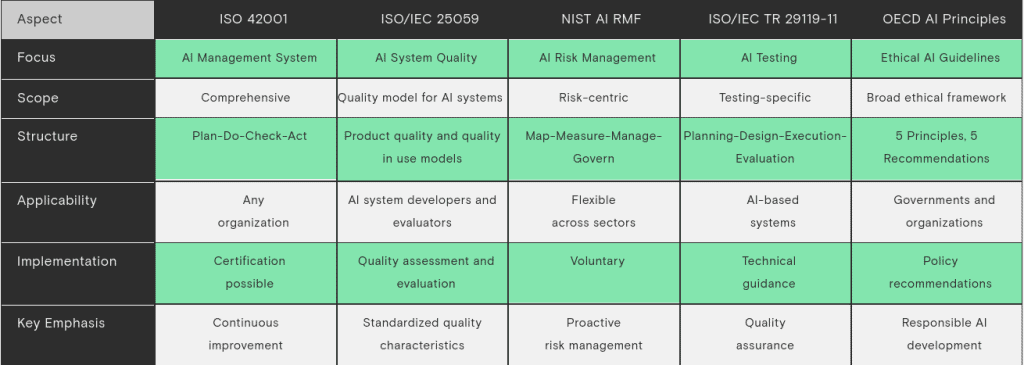
Aspect
Focus
ISO 42001
AI Management System
ISO/IEC 25059
AI System
Quality
NIST AI RMF
AI Risk Management
ISO/IEC TR 29119-11
AI Testing
OECD AI Principles
Ethical AI Guidelines
Scope
Structure
Comprehensive
Plan-Do-Check-Act
Quality model for AI systems
Product quality and quality in use models
Risk-centric
Map-Measure-Manage-Govern
Testing-specific
Planning-Design-Execution-Evaluation
Broad ethical framework
5 Principles, 5 Recommendations
Applicability
Implementation
Any
organization
organization
Certification
possible
possible
AI system developers and evaluators
Quality assessment and evaluation
Flexible
across sectors
across sectors
Voluntary
AI-based
systems
systems
Technical
guidance
guidance
Governments and organizations
Policy
recommendations
recommendations
Key Emphasis
Continuous
improvement
improvement
Standardized quality characteristics
Proactive
risk management
risk management
Quality
assurance
assurance
Responsible AI development
Mapping the Standards to the EU AI Act Requirements
Alignment with the EU AI Act
The four AI standards can be seen as complementary tools for organizations seeking to comply with the EU AI Act.
ISO 42001 aligns with the EU AI Act’s focus on risk management and governance and provides a framework that can support compliance with the Act’s requirements.
NIST AI RMF complements the EU AI Act’s risk-based approach and offers flexible implementation strategies that can be adapted to meet the Act’s requirements.
ISO/IEC TR 29119-11 addresses specific testing needs, supporting the EU AI Act’s requirements for high-risk AI systems while providing technical guidance that can help meet the Act’s standards for quality, reliability, and safety.
The OECD AI Principles are closely aligned with the EU AI Act’s fundamental principles, providing the ethical framework that underpins many of the Act’s requirements.
ISO/IEC 25059 introduces quality characteristics specific to AI systems, supporting the EU AI Act’s emphasis on AI system quality. It offers a standardized approach to quality assessment, which can aid in demonstrating compliance with the Act’s requirements for high-risk AI systems. It also addresses aspects like robustness and transparency, which are key concerns in the EU AI Act.
The following table demonstrates how each standard can help businesses comply with the EU AI Act.
EU AI Act Core Requirement
Risk Management
ISO 42001
Provides a structured framework for managing AI governance, aligning with risk-based compliance.
ISO/IEC 25059
Emphasizes system reliability and adaptability to mitigate risks.
NIST AI RMF
Focuses on identifying, assessing, and mitigating AI-related risks through a systematic approach.
ISO/IEC TR 29119-11
Offers testing protocols to evaluate the robustness and resilience of AI systems
OECD AI Principles
Encourages proactive governance for ethical risk mitigation.
Transparency
Accountability
Promotes transparency through documentation and governance practices.
Embeds accountability in organizational AI management systems.
Establishes metrics for explainability and documentation of decisions.
Ensures systems meet predefined quality benchmarks, reinforcing accountability.
Recommends processes for documenting and communicating risk mitigation activities.
Encourages continuous monitoring and evaluation of AI risks, ensuring ongoing accountability.
Guides in testing explainability features to ensure clear and interpretable outputs.
Supports validation processes to ensure systems meet accountability requirements.
Prioritizes transparency as a core principle for responsible AI use.
Advocates for accountability as a foundational value.
Bias and Fairness
Robustness and Security
Integrates fairness considerations into the governance framework.
Focuses on integrating robust governance systems for secure AI deployment.
Provides metrics to evaluate and address bias in datasets and models.
Defines quality attributes for robustness, ensuring systems operate reliably.
Includes provisions for identifying and mitigating algorithmic bias.
Provides tools for assessing and improving system security and robustness.
Tests for performance across diverse datasets to detect and address biases.
Delivers technical guidelines for stress-testing and validating AI model robustness.
Highlights fairness as an essential component of ethical AI.
Encourages the development of secure and trustworthy AI systems.
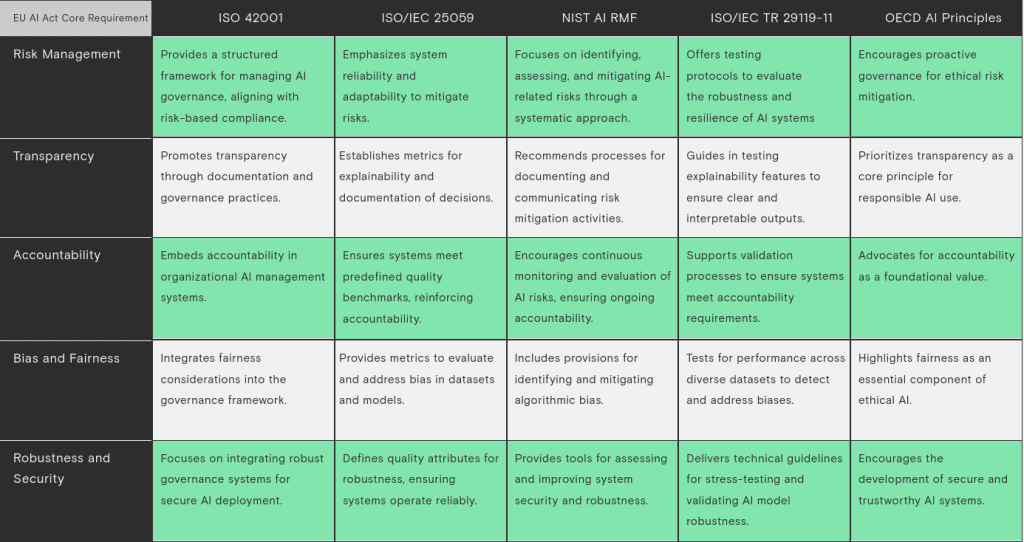
Choosing the Right Standard for Your Business Use Cases
Different business scenarios require distinct AI governance approaches. This section provides guidance on selecting the appropriate standard based on the use case, risk profile, and regulatory context.
For Compliance-Heavy Industries in the EU (e.g., Financial Services, Healthcare)
Recommendation: ISO 42001, ISO 25059, and the EU AI Act.
ISO 42001 ensures a comprehensive governance framework, while ISO 25059 adds quality benchmarks for functionality, reliability, and fairness. Together, they help meet stringent regulatory and ethical demands. The EU AI Act complements these standards by providing risk-based compliance pathways.
For High-Risk AI Applications (e.g., Autonomous Vehicles, Medical Diagnostics)
Recommendation: ISO/IEC TR 29119-11, NIST AI RMF, and ISO 25059.
For systems requiring rigorous performance validation, ISO/IEC TR 29119-11 and ISO 25059 provide robust testing and quality evaluation protocols. NIST AI RMF strengthens this by addressing risk management and resilience.
For Trustworthy AI and Fairness (e.g., Public Sector, Consumer Services)
Recommendation:
OECD AI Principles, ISO 25059, and the EU AI Act.
OECD AI Principles provide the ethical foundation, while ISO 25059 ensures measurable quality and fairness in system design. The EU AI Act enforces these standards with regulatory backing, ensuring compliance with societal expectations.
For Organizations with Broad AI
Governance Needs (e.g., Multinational Corporations)
Recommendation: ISO 42001, ISO 25059, and OECD AI Principles.
These standards combine structured governance (ISO 42001), measurable quality (ISO 25059), and ethical considerations (OECD Principles), providing a holistic toolkit for diverse use cases across regions.
In addition to the specific use cases mentioned above, it is crucial to consider the organization’s size, industry, and risk appetite when selecting an AI standard. Organizations may also choose to adopt multiple standards to address different aspects of AI governance comprehensively.
Note: This guide provides a general overview of AI standards and their application. Organizations should seek expert advice to determine the most appropriate standards for their specific needs and circumstances.
Take the Next Step Toward Responsible AI Governance
At code4thought, we believe that the future of AI lies in its ability to transform industries while upholding the highest standards of trust, transparency, and fairness. Whether you’re navigating compliance with the EU AI Act, striving for operational excellence, or seeking to minimize risks in high-stakes applications, our expertise and solutions are here to guide you every step of the way.
Our AI Quality Testing platform, iQ4AI , is designed to operationalize the principles outlined in this guide. From ensuring robust model performance to mitigating bias and enhancing explainability, iQ4AI simplifies the complexities of AI governance. With advanced tools for testing, evaluation, and reporting, it empowers businesses to align their AI systems with international standards and regulatory requirements seamlessly.
code4thought is your partner in building AI systems that are not only compliant but also trustworthy, reliable, and innovative through its Trustworthy AI services, ranging from AI Quality Testing to regulation-specific NYC Bias Audit and EU AI Act Assurance service, as well as AI Due Diligence.
Ready to transform how you govern and manage AI? Contact our team today.