AI Quality Testing
& Audit
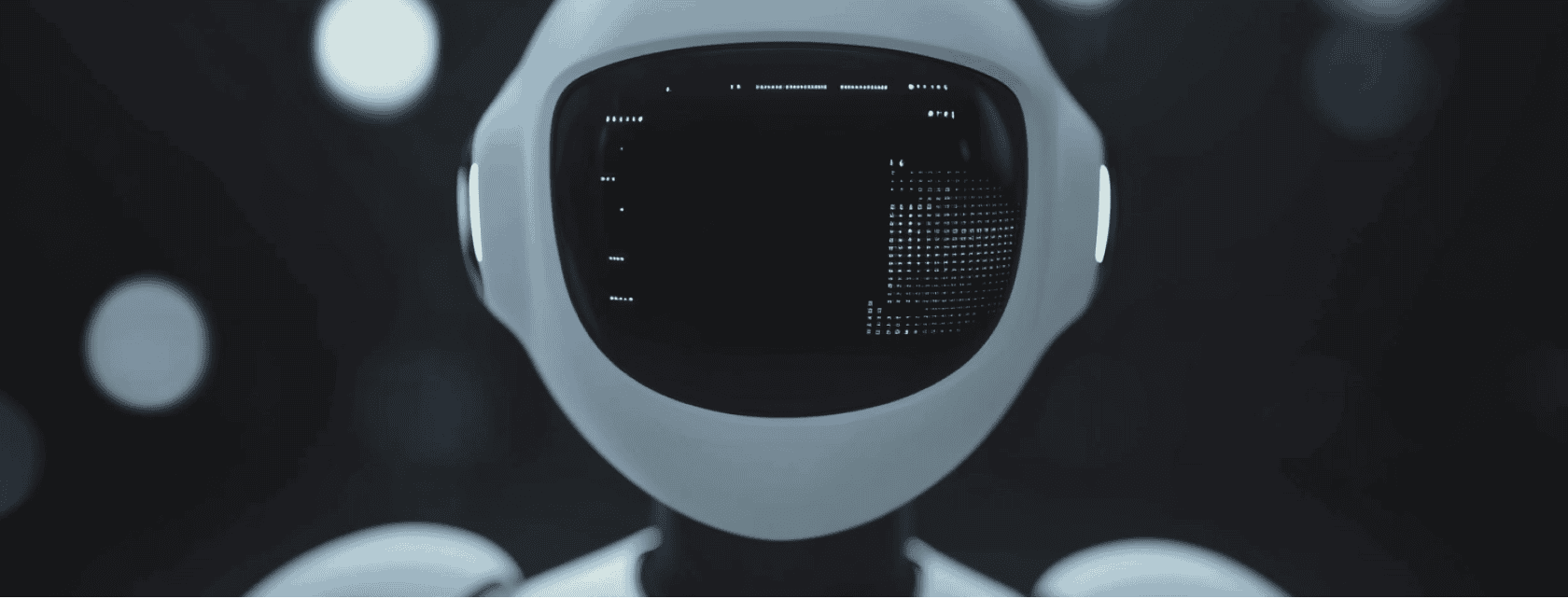
SOLUTIONS >AI TESTING & AUDIT

We offer a well-structured and flexible AI Quality testing and auditing framework, which combines automated tools based on the ISO 29119-11 international standard for testing AI-based systems and human expertise.
Our solution provides useful and actionable insights in a meaningful and efficient manner, into the AI Risk Assessment, AI Governance and Compliance Evaluation processes of an organisation.
It also serves as a comprehensive technical guide for fostering responsible AI deployment and usage, by promoting quality, transparency, accountability and human-centric AI practices within the organization, while maximizing the business value of AI systems.

Identifying complex AI issues at the root
Our experts start an AI Quality Testing & Audit project by first understanding the business (or social) and technical context and then digging deep into the provided dataset(s) and model(s) to perform a comprehensive analysis from different perspectives.
Based on the analysis results, our team works to reveal the root causes of core issues and provide the respective recommendations.
Typically, we test the AI systems from the perspectives of Performance, Fairness/Bias, Transparency, Robustness and Reliability characteristics (or quality aspects) as defined by the ISO 29119-11 standard. These analyses provide the insights needed for improving the behavior and trustworthiness of a given AI-based system.

Ensuring compliance with legislation
We have the flexibility to adopt our AI quality testing & auditing processes to specific requirements, generated either by clients’ needs and/or aiming compliance with existing and upcoming regulations such as the EU AI Act, UK’s framework for AI Regulation and the Blueprint For An AI Bill Of Rights in the USA. Our solutions EU AI Act Assurance and NYC AI Bias Audit are indicative examples.
What we test
Our own proprietary AI Quality testing platform, iQ4AI
enables the analysis of any type of data and AI models based on the ISO 29119-11 international standard for testing AI-based systems.
We dig deep into the AI model and the data it utilizes in order to provide a comprehensive overview of all relevant aspects of an AI system, so that our clients can understand what’s going on in the AI model/algorithm itself.
Type of project: Either one-off or with audits at predefined intervals.
Our analysis focuses on the Quality of the AI system and includes:
Performance Testing
We assess how effectively an AI system performs its intended tasks. This includes evaluating the system’s accuracy and efficiency in various operational scenarios. It’s crucial for determining whether the AI system meets the operational requirements of a business.
Trustworthiness Testing
Τhis type of analysis encompasses several critical aspects.
Fairness / Bias Testing: Ensuring the AI system does not exhibit biased decision-making and treats all user groups equitably.
Transparency / Explainability Analysis: The provision of explanations on how the decisions of the AI system are being derived and assessment of the validity, clarity and understandability of the decision-making processes
Robustness / Security & Safety: Testing for data variance related vulnerabilities to protect the system from cyber threats targeting an AI system’s potential robustness shortcomings and safeguard data integrity.
Reliability: Evaluating the consistency and dependability of the AI system over time and under different conditions.
This bifurcation ensures a comprehensive evaluation of AI systems, addressing both their functional efficiency and their ethical, secure, and transparent operation.
Based on these results, as well as our clients’ own business context, our advisors develop a full AI risk profile and actionable, prioritized recommendations to ensure their objectives stay on track.
Features

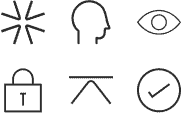
Our comprehensive set of specialized tests and analyses provide deep insight into the state of your AI-system and input for improvement or risk management programs.

Technical
Assessment
Assessment
We evaluate AI systems, algorithms, and models to ensure compliance with technical standards and guidelines. We conduct thorough technical testing using our AI Quality Testing platform, iQ4AI and analysis to identify vulnerabilities and areas for improvement.

AI Risk Assessment
& Mitigation
& Mitigation
We identify potential risks associated with AI deployment and operations. These risks may be associated with data vulnerabilities, and AI assets’ performance, robustness, security, explainability and transparency; they may even have legal implications. They are evaluated and assigned an appropriate “importance level” with a proprietary, intuitive scoring algorithm. Finally, strategies and frameworks are developed to mitigate these risks effectively.

Continuous Monitoring &
Auditing (optional)
Auditing (optional)
We use iQ4AI to perform independent, recurring audits combined with on-going advisory support. Continuously enhance the mechanisms established for the ongoing monitoring, auditing, and reporting processes, to ensure risk mitigation and compliance, as regulations evolve and as the organization’s AI landscape changes.
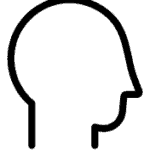
Why us
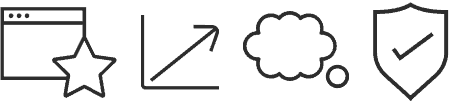
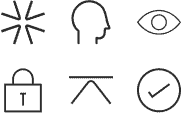
Our AI Quality Testing & Audit solution follows a proven, fact-based method for enhancing the AI strategy and informed decision-making of an organization as well as their ability to mitigate any risks or mistrust related to their AI-based systems.
Partnering with us means:
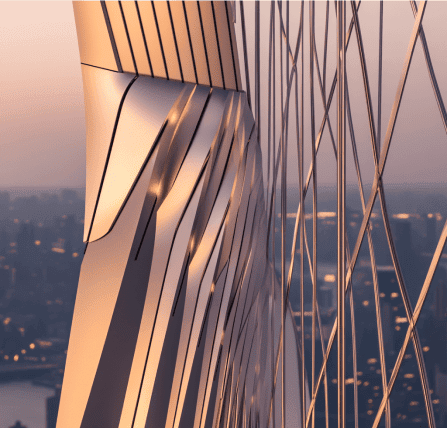
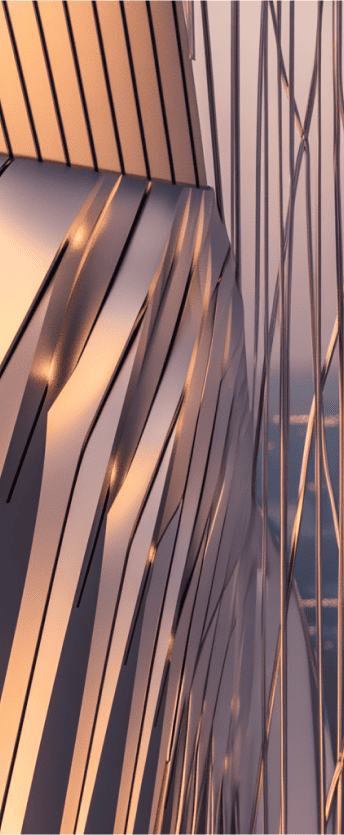
International industry standards and benchmarks,
by following the guidelines of the ISO 29119-11 standard for testing AI-based systems and as imposed by the ISO 42001 and ISO 5338
Industry, Model and Data-Agnostic solutions: we support the analysis of any type of AI-model (from a simple rule-based to a deep learning one) and data (i.e. from credit risk datasets to image and video data). In that way we are flexible enough to help organizations across different business domains, from healthcare and high-tech to telecoms, banking and government.
Root-cause analysis: Mitigating risks related to the behavior of an AI-system requires a proper factual and data-driven analysis that brings all facts to the table. Our expert advisors audit your AI-system to get to the core of any issue. Our fact-based reports will align your organization to address the root causes immediately.
Following the Human-in-the-Loop principle: This approach is essential in all phases of AI system development and operation and when ethical and subjective assessments are required. It ensures a comprehensive understanding of AI decisions, particularly in complex, high-stakes environments.
Independent, objective advisory, so no strings attached with AI vendors or big tech companies:
Our team provides advice and recommendations that are impartial and objective. We have no stake in the outcome and focus only on the facts and identifying those issues that can create problems in the short-term as well as long-term.
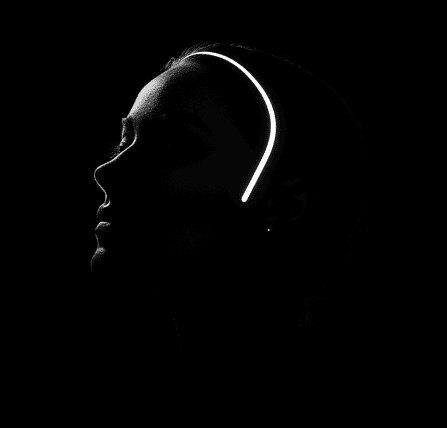
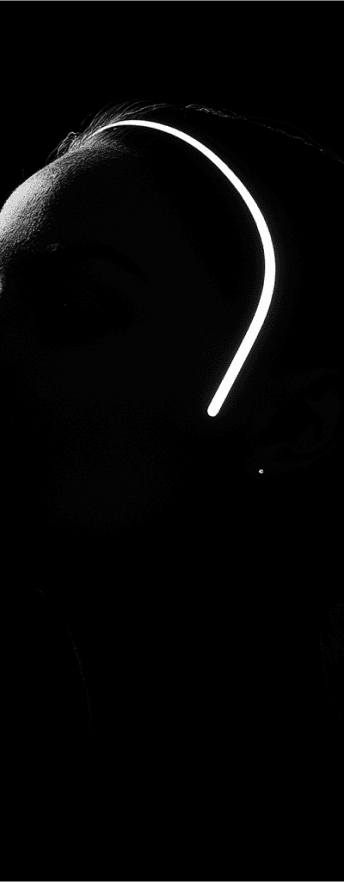
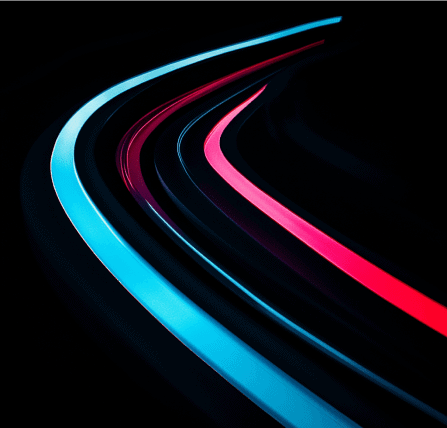
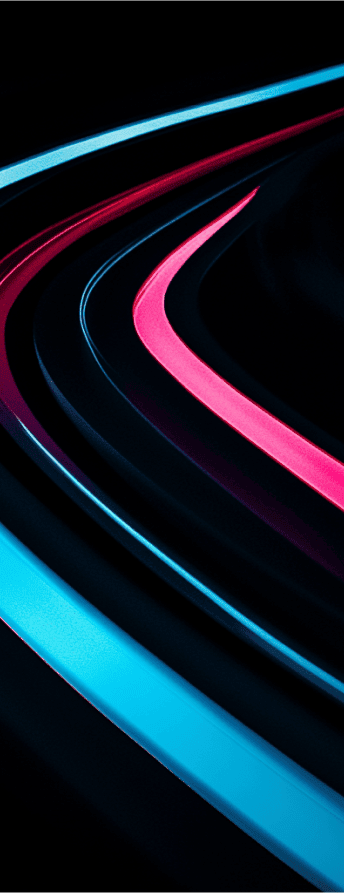
Pragmatic and actionable suggestions for improvements, so no theoretical or out of context advice: Our guidance and recommendations lead to measurable improvements through our iQ4AI AI testing platform. That means you can start your improvement plan right away alongside our guidance and advisory.
Client-specific approach: Our services are tailored to the unique requirements and circumstances of each organisation, so as to develop comprehensive compliance strategies, risk mitigation and implementation plans, aligned with the client’s business objectives, industry sector, and regulatory requirements.
Cross-Disciplinary Expertise: our team combines legal, technical, and ethical perspectives on AI governance. By assembling a team of experts with diverse backgrounds and skill sets, code4thought provides solutions that address the multifaceted challenges of the AI domain.
Unique combination of expert advisory and proprietary AI testing platform, iQ4AI : our capabilities can support accuracy, speed, consistency and continuity on AI Risk Management frameworks being implemented at scale.
Proven Track Record: code4thought has an extensive track record in assessing risks associated with large-scale software systems across various industries and sectors. Our experts are (more than) capable in identifying, analysing, and mitigating complex risks inherent in software development and deployment. Such experience offers valuable insights and best practices that are also applicable to AI systems operating in diverse contexts.
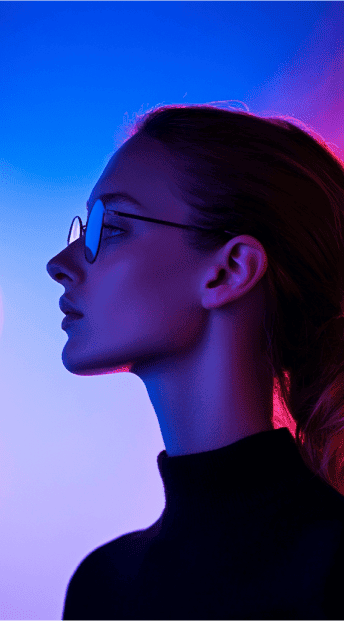
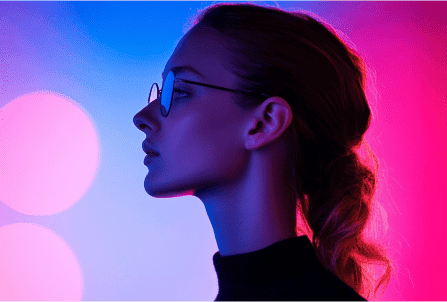
Benefits

Maximize Business Value & Improve ROI
Technical assessment ensures that AI systems perform as intended under various conditions, leading to improved efficiency and productivity. By optimizing performance and reliability, organizations can achieve better outcomes and maximize the value derived from their AI investments.
Periodical monitoring & auditing ensures that each AI asset always represents and resolves a current business problem. If not, it facilitates timely corrections and enhancements
A well-designed monitoring and continuous learning process is key to adapting to evolving regulatory requirements and industry standards and maximizing the business value any AI asset may add over time.

Risk Mitigation
Identify and address legal, ethical, and reputational risks associated with AI deployment, safeguarding organisation.

Enhance Governance & Build Trust
Demonstrate your commitment to responsible AI practices, enhancing confidence and trust among regulatory authorities, customers, investors, and other stakeholders.

Compliance Assurance with minimum adaptation costs
Gain assurance that your organization meets the regulatory requirements and obligations, mitigating the risk of non-compliance penalties and legal issues.
At the same time, our comprehensive solution contributes to reducing the initial costs required for timely compliance as well as subsequent costs, by minimising the risk of costly and hasty implementations on the client side which may add technical debt due to lack of adequate analysis & planning.


Maximize Business Value & Improve ROI
Technical assessment ensures that AI systems perform as intended under various conditions, leading to improved efficiency and productivity. By optimizing performance and reliability, organizations can achieve better outcomes and maximize the value derived from their AI investments.
Periodical monitoring & auditing ensures that each AI asset always represents and resolves a current business problem. If not, it facilitates timely corrections and enhancements
A well-designed monitoring and continuous learning process is key to adapting to evolving regulatory requirements and industry standards and maximizing the business value any AI asset may add over time.

Risk Mitigation
Identify and address legal, ethical, and reputational risks associated with AI deployment, safeguarding organisation.

Enhance Governance & Build Trust
Demonstrate your commitment to responsible AI practices, enhancing confidence and trust among regulatory authorities, customers, investors, and other stakeholders.

Compliance Assurance with minimum adaptation costs
Gain assurance that your organization meets the regulatory requirements and obligations, mitigating the risk of non-compliance penalties and legal issues.
At the same time, our comprehensive solution contributes to reducing the initial costs required for timely compliance as well as subsequent costs, by minimising the risk of costly and hasty implementations on the client side which may add technical debt due to lack of adequate analysis & planning.
FURTHER READING
The Quality Imperative: Why Leading Organizations Proactively Evaluate Software and AI Systems with Yiannis Kanellopoulos, hosted by George Anadiotis
In an increasingly AI-driven world, quality is no longer just a technical metric—it's a strategic imperative. In this episode of...
Read MoreISO 42001 Advisory Form -Evaluate Your Readiness for Responsible AI Governance
As AI systems become more central to business operations, the need for formalized, accountable, and ethical AI practices has never...
Read Morecode4thought at Two Major International AI Conferences This April
We are proud to announce that our CEO, Yiannis Kanellopoulos, will be representing code4thought at two prominent international events this...
Read More