NYC AI Bias Audit
NYC AI Bias Audit Law Solution
Reliable AI
Βias Τesting
- Disparate impact analysis on persons (e.g., candidates, employees)
- Per protected categories (e.g., gender, ethnicity, race)
Findings Report with Mitigation Measures
lorem ipsum lorem ipsum lorem ipsum
Summary of Results for publishing
lorem ipsum lorem ipsum lorem ipsum lorem ipsum lorem ipsumlorem ipsumlorem ipsum
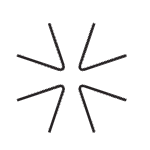
Why us?
NYC Local Law 144 Summary
Bias audit
Published Results
Notice to Candidates
Penalties for non-compliance
Frequently Asked Questions
- They use an automated employment decision tool (e.g., resume screening) whose output such as a score, classification or recommendation is used
- To evaluate candidates or employees
- Seeking a position or promotion
- And are residing in New York City (this also includes remote work positions).
- to rely solely on a simplified output (score, tag, classification, ranking, etc.), with no other factors considered; or
- to use a simplified output as one of a set of criteria where the simplified output is weighted more than any other criterion in the set; or
- to use a simplified output to overrule conclusions derived from other factors including human decision-making.
- that generate a prediction, meaning an expected outcome for an observation, such as an assessment of a candidate’s fit or likelihood of success, or that generate a classification, meaning an assignment of an observation to a group, such as categorizations based on skill sets or aptitude; or
- for which a computer at least in part identifies the inputs, the relative importance placed on those inputs, and, if applicable, other parameters for the models in order to improve the accuracy of the prediction or classification.
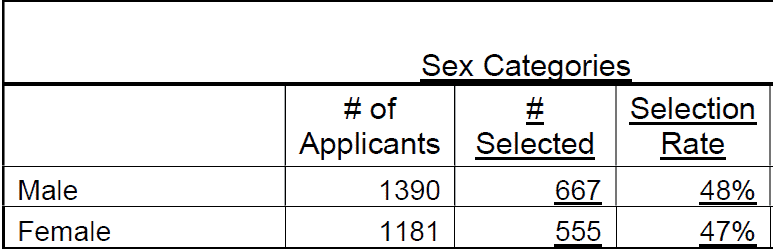
Example
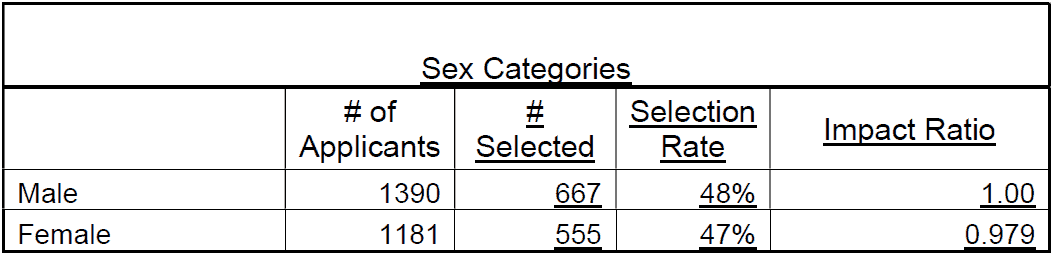
Selection/Scoring rate and Impact Ratio must separately calculate the impact of the AEDT on:
- Sex categories
i.e., impact ratio for selection of male candidates vs female candidates, - Race/Ethnicity categories
e.g., impact ratio for selection of Hispanic or Latino candidates vs Black or African American [Not Hispanic or Latino] candidates - intersectional categories of sex, ethnicity, and race
e.g., impact ratio for selection of Hispanic or Latino male candidates vs. Not Hispanic or Latino Black or African American female candidates.
Example
Τhe number of individuals the AEDT assessed that are not included in the calculations because they fall within an unknown category, should be mentioned in a respective note in a the summary of results.
Example Note: The AEDT was also used to assess 250 individuals with an unknown sex or race/ethnicity category. Data on those individuals was not included in the calculations above.
- not be involved in using, developing, or distributing the AEDT
- not have an employment relationship with the employer or the employment agency or the AEDT software vendor at any point during the bias audit or
- have no financial interest in the employer or the employment agency or the AEDT software vendor at any point during the bias audit
This Phase (Analysis Phase) usually takes 1-3 weeks depending on the Project.
The Reporting Phase follows, during which code4thought prepares the results, which are presented to the Client for validation and finally we have the final report session.
Reporting Phase usually takes 1-3 weeks, as well.
WE' D LOVE TO HELP YOU
WE' D LOVE TO HELP YOU
WE' D LOVE TO HELP YOU
WE' D LOVE TO HELP YOU
WE' D LOVE TO HELP YOU
WE' D LOVE TO HELP YOU
Let's get started with your AI Bias Audit!
Let's get started with your
AI Bias Audit!
FURTHER READING
OECD AI Principles: Guardrails to Responsible AI Adoption
Amidst the transformative wave of artificial intelligence (AI) invoked in the digital ecosystem, the Organization for Economic Cooperation and Development...
Read MoreA Technical Report Providing Guidelines for Testing AI-Based Systems
Today, software permeates every aspect of life, and artificial intelligence (AI) is becoming increasingly integral to business operations. As AI...
Read MoreOWASP Top 10 Vulnerabilities for Large Language Models (LLM): Impact and Mitigation
Large Language Models (LLMs) have garnered significant attention since the mass-market introduction of pre-trained chatbots in late 2022. Companies keen...
Read More