The success story of QUALCO’s
investment in an AI/ML startup
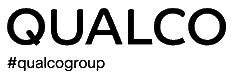
CASE STUDIES > AI DUE DILIGENCE
- The Challenge
QUALCO is a leading technology company that provides a wide range of data-driven solutions to help enterprises efficiently manage customers and assets. Recently, the company was considering acquiring an AI startup and needed a trusted partner to conduct the Due Diligence process and assess the company’s assets in a fact-based and independent way.
To meet this challenge, QUALCO turned to code4thought, an experienced and trusted partner with a proven track record in conducting AI DDs. Through its thorough evaluation, code4thought helped QUALCO gain visibility into the following:
The current state of the startup’s inventory of AI systems
The team’s maturity in terms of systems’ development, governance and ability to mitigate any potential technical risks
The systems’ lineage and the safety and performance testing they have undergone
- The Solution
Our team successfully executed the AI Technology Due Diligence project for QUALCO within six (6) weeks following the below process:
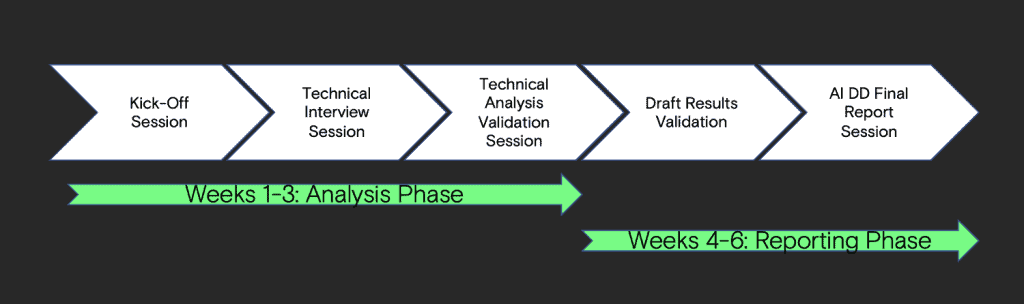
Firstly, we aligned expectations with both QUALCO, and the startup’s team with whom we were to perform Due Diligence. This included verifying the scope of work, the client’s research questions and agreeing on the project’s process and timeframe.
Once the scope of work and research questions were defined, we continued with the information-gathering stage, which included:
- provided documentation
- custom-made questionnaires based on proven and accepted industry-standards
- the respective validation dataset for the system in scope for the testing,
- interviews with the founding team of the AI startup
Our AI Maturity Governance questionnaire consists of 6 main sections that involve:
- General questions
- Intended use
- Domains and applications
- Performance
- Safety
- Concept drift
- Explainability and fairness
- Monitoring
- Security
6. Lineage
Additionally, to get in-depth information about the maturity of their MLOps procedure and assess if they apply some widely-accepted best practices, the startup’s team was asked about the following:
- ML metadata tracking and logging
- Continuous
- Integration/Delivery (CI/CD)
- Monitoring
- ML evaluation
3. MLOps Workflow orchestration
4. Reproducibility
5. Versioning of data, code and model
6. Collaboration between the members of the team
7. Feedback loops that are present in each step of the procedure
AI system Testing
An inseparable part of our AI Technology Due Diligence service is the dynamic testing of an AI system. In this project, we tested a key AI system of the startup’s AI inventory for its proper implementation, timely deployment and reliable operation. This system was performing a binary classification of the criticality of alarms and a multiclass classification of the system/subsystem of a new alarm tag. It was generating the most revenue for the startup, already operational in a portfolio of their clients. To test the AI model, we were granted access to it via a REST API.
The startup provided a testing/validation dataset to test the system’s performance and identify potential bias issues. This dataset was given as input to the AI model via calls on a REST API that the startup has granted us access to. The API returned as a response the predictions of the model, specifically the predicted criticality (critical or non-critical) and the predicted system/subsystem of an alarm tag.
Two main types of tests were performed:
1. Performance tests
We checked the model’s performance using 3 different evaluation metrics (precision, recall, f1-score). Additionally, we calculated the performance per class to identify underperformance. First, we checked the criticality class, where no significant issues were detected, except for a slight problem in the precision score of the critical class compared to the non-critical one.
Next, the system/subsystem class was checked, and our analysis pointed out that one of the four systems in the dataset had lower performance than the others. Overall, the model performed relatively well across all evaluation metrics.
2. Bias tests
Bias tests focused on detecting potential statistical bias issues, given that the startup primarily dealt with Industrial AI and machinery and did not directly impact the assets or human operators. To detect potential statistical bias issues, our approach was to evaluate the performance between different groups (the unique values of the criticality class were selected) and subgroups (the 4 different systems were selected).
Our analysis revealed a statistical bias issue where one of the systems’ performance significantly differed between critical and non-critical classes across all the evaluation metrics.
- Results & Outcomes
After completing the information processing and testing, we organised a session with the AI startup’s engineering team to validate our analysis findings. The main insights upon this validation were:
- Human-in-the-loop was a key concept for the startup
- There was recency in the startup services latest releases
- Their system falls into the limited risk category as defined by the upcoming EU AI Act
- There is no mitigation plan when it comes to a security breach
- There was a lack of orchestration mechanisms for the ML procedure
- The startup did not follow the standard practices as per the versioning of the model artefact, and the model predictions
- The appropriate metrics are being used for the evaluation of the performance of their AI-based services
After validating all aspects of the AI startup based on the ISO-29119-11 standard, our team identified strengths and areas for improvement. We provided recommendations and a high-level improvement roadmap. Our analysis yielded the following outcomes:
- The startup is using state-of-the-art technologies and frameworks to build its models ,
- Controls and monitors for most of the crucial operations (e.g. checks for statistical bias) of their AI-based services are defined and operationalised,
- All AI models exhibit a basic level of transparency, as defined in the ISO-29119-11 standard, which ensures that users are aware they are interacting with an AI system.
- The startup is using appropriate metrics to measure the performance of its AI-based services.
- Benefits
With our solution, QUALCO gained a better understanding of the following:
– Whether the startup’s team is mature enough to build accountable and trustworthy AI systems.
– The improvement areas of the startup and a clear path to go.
As a result, QUALCO has now:
– All the appropriate insights to assess the potential investment in the startup’s technology
– Clear recommendations to mitigate potential issues caused by the model’s decisions
– Increased alignment and specific expectations on the technology across multiple levels and stakeholders
“Our company was looking to acquire an AI startup to enhance our technology offerings, but first, we wanted to know the potential risks and liabilities. The AI Due Diligence project conducted by the team at code4thought was incredibly thorough. It provided a comprehensive analysis of the AI system, including its strengths and weaknesses, potential risks, and compliance with regulations. Thanks to their insights and recommendations, we can confidently proceed with the acquisition, knowing that we had thoroughly vetted the technology and ensured that it met our high governance, reliability, and performance standards.”
– John Gikopoulos, Chief Innovation Officer | Head AI & Analytics at QUALCO
FURTHER READING
Advanced AI Quality Testing: Ensuring Robust and Trustworthy AI Systems
As AI systems become integral across industries, ensuring reliability, fairness, and trustworthy performance is critical. From healthcare and finance to...
Read MoreThe Ultimate Guide to AI Governance Standards
As AI transforms industries and reshapes business landscapes, organizations face a dual challenge: harnessing its immense potential while ensuring responsible...
Read MoreEnsuring Fairness in AI-Assisted Hiring: Lessons Learned from the NYC Bias Audit Law
As businesses increasingly leverage AI for streamlined recruitment due to its ability to quickly sift through vast applicant pools, a...
Read More