Kepler Vision Technologies –
Building Trust in an AI Healthcare solution
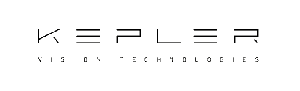
CASE STUDIES > AI AUDIT
This case study was co-written with Olivia Gambelin, AI Ethicist & Author, is based on the Value Canvas, the holistic management template for developing Responsible AI strategies and published at www.thevaluescanvas.com
- The Challenge
Kepler Vision Technologies is a Netherlands-based company with extensive experience in computer vision and artificial intelligence (AI). The company primarily serves the healthcare industry and develops software solutions to improve the efficiency of healthcare delivery while also maintaining the safety and well-being of customers and patients.
Its innovative Kepler Night Nurse AI application is particularly pivotal in scenarios such as fall prevention and detection and monitoring patients for wandering or other undesirable behaviors. The tool is critical in environments like healthcare facilities and hospitals where patient safety is paramount.
Building Trust in AI For Healthcare at Scale
In the highly regulated healthcare industry, gaining and maintaining trust in AI-driven systems is a significant challenge. Kepler recognizes that when entering new markets or engaging with new clients, it is imperative not only to prove that their software has been rigorously tested, but also to demonstrate effective governance over the development and deployment of these technologies.
To navigate these complexities, Kepler partnered with code4thought, acknowledging our proficiency in assessing and testing AI systems using a proprietary software platform. code4thought’s role extends beyond mere testing; it provides a crucial governance mechanism, ensuring that AI applications meet the highest regulatory compliance standards, like the EU AI Act, and operational integrity. Our proprietary platform is instrumental in this process, offering robust tools for auditability, traceability, and verification of AI technologies.
The collaboration between Kepler and code4thought exemplified a successful approach to integrating testing and auditing technologies in sensitive and regulated environments like healthcare. This integration involved a comprehensive assessment methodology that includes:
- Validation and Verification: rigorous testing to validate the effectiveness of AI algorithms and verify their performance according to the given context (healthcare) and problems at hand.
- Risk Management: systematic approach implementation to identify, assess, and mitigate risks associated with AI deployment in healthcare settings.
- Technical Audits: Technical audits, to ensure AI systems’ quality and trustworthiness meet the highest possible standards (as also defined in regulations and standards).
- Industry Benchmarking: In several cases, we benchmark the AI systems against specific industry standards to ensure they meet the performance criteria necessary for the respective sector.
Kepler’s Needs:
- Short-term: Test the current version of their AI system and identify points for improvement. If possible/necessary fix the most crucial of them.
- Mid-term: Improve their system based on the outcomes of previous analysis
- Long-term: Make testing a standard part of the process/pipeline when implementing their AI system.
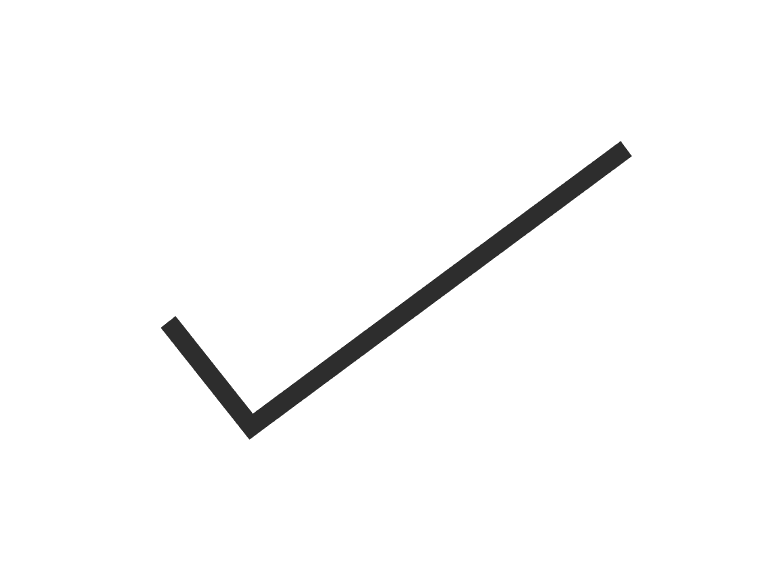
- The Solution
Kepler knew that if it wanted to continue to find success in AI, it needed to standardize its approach to AI governance in a way that was auditable, traceable, and repeatable throughout the company at scale. In other words, Kepler was in need of an Instrument solution. Instrument is the third element of the Process pillar in creating responsible AI, which means the solution for this element needed to standardize execution, automate processes where appropriate, and increase efficiency of Responsible AI governance practices at scale.
code4thought’s proprietary technology, plays a pivotal role in evaluating and ensuring the trustworthiness of the AI models developed by Kepler Vision for critical applications in the healthcare industry. Using the platform to scrutinize the Kepler Night Nurse application’s trustworthiness level by discovering possible model biases and explaining model predictions illustrates a sophisticated approach to addressing two of the most pressing concerns in AI deployment: Bias and Explainability.
Bias Testing
The Bias Testing component of the platform is engineered to detect and quantify unwanted patterns and potential biases in AI models. This mechanism utilizes industry (and regulatory) accepted metrics such as the Disparate Impact Ratio and Conditional Demographic Disparity. These metrics are crucial for evaluating the input and output data used by AI systems to ensure decisions do not unfairly disadvantage any particular demographic group.
In the platform’s deployment for Kepler Vision, the Bias Testing mechanism assessed the AI model’s decisions across different demographic groups. The testing revealed that the decisions made by the AI were free from significant biases, affirming the model’s suitability for diverse and equitable healthcare applications. This outcome was a testament to the maturity of Kepler Vision’s AI development practices and highlighted the effectiveness of the platform’s Bias Testing capabilities in real-world scenarios.
Explainability Analysis
On the explainability front, the platform incorporated a mechanism based on a proprietary algorithm. This algorithm is model-agnostic and is designed to provide clear insights into how AI systems arrive at their conclusions. By analyzing both the input and output data of Kepler’s AI system, code4thought’s platform was able to identify which features were most influential in the model’s decision-making processes. This level of transparency is essential for developers and users alike, as it helps demystify the operations of complex AI systems and fosters greater trust and confidence in their functionality.
The insights gained from the explainability analysis enabled Kepler’s developers to better understand the inner workings of their AI models. This understanding is crucial for ongoing development and refinement, ensuring that the AI systems not only perform effectively but also continue to do so in a manner that is understandable and justifiable to users and regulatory bodies.
Deployment Complexity
The deployment of code4thought’s platform presented unique challenges, particularly in adapting the technology to meet the stringent needs of Kepler Vision. One of the primary concerns was compliance with strict data privacy regulations, which stipulated that sensitive data could not be transferred off-site. The platform was deployed directly on Kepler Vision’s premises to address this, ensuring that all data analysis occurred locally and complied with all regulatory requirements.
This local deployment strategy was also important for the Bias Testing component. Although the evaluation process itself was relatively straightforward, it benefited enormously from being able to operate directly within Kepler Vision’s data environment. This facilitated compliance with data privacy laws and ensured that the bias testing was as relevant and accurate as possible, based on up-to-date and complete data sets.
The Solution’s Assessment
The implementation of code4thought’s platform in evaluating Kepler Vision’s AI system underscores the critical role of proprietary technologies in advancing AI ethics and governance. By integrating sophisticated tools for bias testing and explainability analysis, the platform helps ensure that Kepler Vision’s AI system is not only effective, but also fair and transparent. These attributes are particularly important in the healthcare sector, where decisions made by AI can have significant implications for patient care and outcomes.
Moreover, the collaboration between Kepler Vision and code4thought through the deployment of the platform highlights a model for other AI-driven industries. It demonstrates how AI technology can be deployed in a manner that respects privacy concerns, meets regulatory standards like the EU AI Act, and still provides critical insights that drive better, more trustworthy AI applications.
- The Outcome
Integrating code4thought’s technology into Kepler’s AI development processes marked a significant milestone in enhancing the trustworthiness and quality of AI applications in healthcare. Bias Testing and AI Explainability mechanisms were effectively operationalized, operationalizing essential audits for bias detection and ensuring transparency in the AI decision-making processes for Kepler’s Night Nurse model.
Implementation and Action
The deployment of the platform’s Bias Testing mechanism allowed Kepler to evaluate their Night Nurse model for any unwanted biases systematically. This was crucial, given the diverse patient demographics and the high stakes involved in healthcare outcomes. The Disparate Impact Ratio and Conditional Demographic Disparity metrics applied to the Night Nurse model’s data inputs and outputs ensured that the decisions made by the AI system were free from significant biases. This rigorous testing ensured that the Night Nurse model did not perpetuate existing inequalities or introduce new biases into healthcare decision-making processes.
At the same time, implementing the Explainability mechanism using code4thought’s proprietary algorithm transformed the transparency of Kepler’s Night Nurse model. This mechanism gave developers and stakeholders a clear understanding of how the AI system made decisions. By identifying the features that most significantly influenced the model’s decisions, the deducted explanations provided actionable insights into the Night Nurse model’s operations, enabling ongoing improvements and refinements.
Feedback and Indicators of Success
The feedback from the deployment of code4thought’s platform was positive. Kepler’s engineers reported greater confidence in AI-driven decisions, appreciating the clarity and assurance provided by the explainability as well as the bias testing features respectively. This, helped foster trust and facilitated a broader acceptance of the AI system within the healthcare facility.
Regulators and compliance officers also responded favorably, as the detailed bias audits and transparent decision pathways greatly simplified the compliance verification process. This was especially important as Kepler worked towards getting the Night Nurse model certified as a medical device, which demands stringent adherence to regulatory standards, such as the EU AI Act.
Resolving Core Challenges
Before implementing code4thought’s solution, one of the fundamental challenges faced by Kepler was the skepticism surrounding AI in critical healthcare decisions, primarily due to fears of inherent biases and the opaque nature of some AI operations. code4thought’s solution effectively addressed these concerns by embedding safeguards and oversight mechanisms into Kepler’s Night Nurse system.
The structured risk assessment capabilities provided by code4thought’s platform allowed Kepler to identify and mitigate potential risks and to demonstrate these capabilities to partners and regulators. This structured approach to risk assessment was crucial for navigating the complexities of AI in healthcare, ensuring that all potential issues were addressed proactively.
By leveraging code4thought’s experience and tools, Kepler effectively addressed the dual challenges of innovation and compliance in AI. The case of Kepler highlights the importance of third-party validations and the need for continuous governance to meet legal and ethical standards and ensure that these technological advancements truly enhance patient care and safety.
“As the gravity of decisions made by AI systems increases, so does our need to ensure they operate fairly and transparently. Nowhere is this needed more than in the medical device space, where the judgments of AI powered tools can literally be a matter of life and death. The EU Commission’s proposal for AI systems Regulation makes it clear that more can be done by companies using Deep Learning algorithms with high complexity and opacity to build confidence in AI systems. By working with code4thought, Kepler Vision is confirming its dedication to improving the lives of all patients its technology is applied to, regardless of individual differences.”
– Dr. Harro Stokman, CEO of Kepler Vision Technologies
FURTHER READING
The Quality Imperative: Why Leading Organizations Proactively Evaluate Software and AI Systems with Yiannis Kanellopoulos, hosted by George Anadiotis
In an increasingly AI-driven world, quality is no longer just a technical metric—it's a strategic imperative. In this episode of...
Read MoreISO 42001 Advisory Form -Evaluate Your Readiness for Responsible AI Governance
As AI systems become more central to business operations, the need for formalized, accountable, and ethical AI practices has never...
Read Morecode4thought at Two Major International AI Conferences This April
We are proud to announce that our CEO, Yiannis Kanellopoulos, will be representing code4thought at two prominent international events this...
Read More